By launching 15 new research projects, EUROfusion is engaging data science experts across Europe to apply Artificial Intelligence and Machine Learning techniques to fusion energy. DIFFER researcher Sven Wiesen will be working on the project ‘Machine learning accelerated Scrape Off Layer simulations: SOLPS-NN’.
Fusion energy promises to deliver safe, sustainable, and low-carbon baseload power, complementing other clean energy sources like solar and wind. To achieve this, we need to address complex physics and engineering challenges, including understanding the collective movements of charged particles in magnetic fields, mitigating disruption events, analysing material erosion effects, and processing data rapidly enough for use in control loops. Artificial Intelligence and Machine Learning offer new opportunities to deepen our understanding of these phenomena.
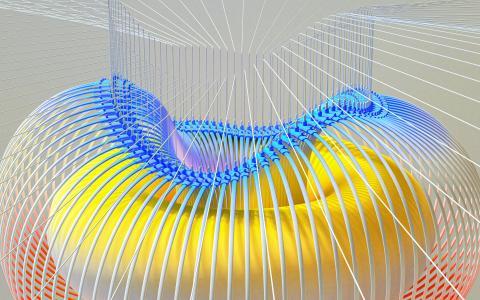
Building on the world's largest dataset
"With new research projects on Artificial Intelligence and Machine Learning, EUROfusion aims to accelerate progress towards fusion energy and support the ongoing efforts in its work packages", explains Sara Moradi of the EUROfusion Programme Management Unit. "Machine learning and Artificial Intelligence are powerful tools for extracting insight from data, uncovering patterns and suggest control schemes that are too computationally intensive to identify with traditional computer models."
EUROfusion’s extensive dataset of fusion experiments spans decades of research, from the earliest fusion machines to the most advanced systems currently in operation. This unparalleled resource positions EUROfusion uniquely to drive forward Artificial Intelligence applications in fusion research.
DIFFER’s contribution
At DIFFER Sven Wiesen, group leader Integrated Modelling, will be working on the project ‘Machine learning accelerated Scrape Off Layer simulations: SOLPS-NN’. Wiesen: "Scrape-off layer simulations for fusion devices take long simulation times as the required model setup must include multi-spatio-temptoral multi-physics components in 2D/3D. After previous successes to speed-up the SOLPS-ITER code into a neural-network version (SOLPS-NN, EUROfusion ENR work) we want to bring the fast edge modelling to another level, by including more physics and dynamics into the fast SOL model through modern ML/AI techniques like transfer- and active-learning."
Artificial Intelligence for Fusion projects
Following a call and selection process, the EUROfusion General Assembly approved the support for 15 new Artificial Intelligence Fusion research projects. The strong response to the call highlights the scientific community's commitment to using state-of-the-art approaches to advance computational techniques for magnetically confined plasmas. The 15 projects will receive a total amount of €2.659 million, of which half is provided by collaborative co-funding from the researchers' home institutes and half from EUROfusion. The research projects will run for a period of two years.
About EUROfusion
The EUROfusion consortium coordinates experts, students and facilities from across Europe to advance fusion energy in line with the EUROfusion fusion roadmap. Co-funded through the Euratom Research and Training Programme, EUROfusion supports the preparation for experiments at ITER and the development of the European demonstration fusion power plant DEMO. The programme also fosters fusion education, training, and industry collaboration.
Author: EUROfusion, DIFFER
Go to the News page.